1. INTRODUCTION
Dengue fever is one of the world’s most important neglected tropical diseases, with the number of cases increasing 30-fold in the last decades(1). It is estimated that every year worldwide there are about 390 million dengue virus infections, of which about 500,000 develop into severe form and there are more than 25,000 deaths (1). According to the World Health Organization (WHO), in 2009 in Vietnam, as of the 19th week the number of suspected cases reported was 59,959, including four deaths three times higher than the same period in 2018(2). According to the statistics of Ministry of Health, Ba Ria - Vung Tau province has the increasing number of dengue cases per week, and the number of accumulated cases, also it number of cases per 100,000 people is highest over the country (3).
Vietnam has a humid monsoon climate that is not homogeneous throughout the territory, forming regions and regions with markedly different climates. Increased population density accompanied by increasing urbanization, polluted environment, climate change contributes to an increase in dengue cases in the community (4, 5). In particular, weather factors (rainfall, temperature, humidity, etc.) have affected the number of dengue cases. Recently, there have been many studies building a model for forecasting dengue based on weather factors; models used to build forecasting models such as Poisson regression model, SARIMA model, GAM model, etc. It is commonly used in the world and Vietnam (6-10). The artificial neural network (ANN) model is a new approach in identification and forecasting, receiving special attention from researchers around the world, the ANN is considered a powerful tool to solve problems that are non-linear, complex, and cases where relationships are difficult to establish. Around the world, the study by Napa Rachata and colleagues used the ANN to predict dengue outbreaks, with a highly accurate prediction of up to 85.92%(11); Another study by author Jorge D. Mello-Román and colleagues comparing two methods, ANN and assisted vector (SVM) in diagnosing dengue, found that the ANN (accuracy: 96%) had better forecast results than the SVM method (accuracy: 90%)(12). In Vietnam, the study uses the ANN model to predict largely in the field of economics and electronic (13-15), etc. There are very few studies using ANN in the field of preventive medicine, namely the forecast of the dengue epidemic.
Vietnam is an epidemiological region of dengue, besides, there is currently no vaccine against dengue. In our country, the common measures to prevent dengue are to kill mosquitoes, mausoleums/sticks and mosquito bite prevention has worked well (16). At the same time, the establishment of a dengue early warning system to improve the ability to predict outbreaks remains an important step in controlling dengue outbreaks. Therefore, we conducted this study to build an ANN model to forecast the dengue epidemic based on weather factors in Vung Tau City in Ba Ria - Vung Tau province.
2. MATERIALS AND METHOD
Collect data on all cases of dengue in Vung Tau City in Ba Ria - Vung Tau province in the period of 1/2010 – 12/2020.
Data on weather factors, including temperature (°C), precipitation (mm), humidity (%), wind speed (m/s) in Vung Tau City in Ba Ria – Vung Tau province in the period of 1/2010 – 12/2020.
Time-series study. Ecological study, and the data were taken from Jan 2010 to Dec 2020. A time series was use to assess the association between weather conditions (i.e., temperature, humidity, rainfall, and wind speed) and dengue cases, using the ANN model to forecast the number of dengue cases.
Implementation time: 01/2010 – 12/2020.
Location: Vung Tau City of Ba Ria - Vung Tau Province.
Consider the correlation between variables:
The Speaman’rank test is used to check the correlation between weather variables and dengue case variables.
ANN model building:
Network architecture: In this study, we predicted the time series using a reverse propagation neural network (which is a network with a straight transmission structure, supervised learning, and the use of a reverse propagation algorithm) consisting of 3 layers (in, hidden, and out-of-class). Our study used data on dengue cases and weather factors (temperature, precipitation, humidity, wind speed) of 132 months (11 years) to build a dengue forecast model. ANN model is built in 5 steps:
Step 1: Collect and process data
Data on dengue cases and monthly weather factors in the period 1/2010 - 12/2020.
The collected data set will be divided into two sets of sub-data: 120 months (period 1/2010 - 12/2019) used to train the ANN and 12 months (1 - 12/2020) used to check the ANN to determine the reliability of the forecast model.
Step 2: Build an ANN structure (13)
A number of hidden layers: Typically, the design of the ANN will start with a hidden layer. If the number of neurons is too large (>50) and the error is still unacceptable, increase the hidden layer to 2. This process is repeated until the desired error and output are achieved.
A number of hidden neurons (neuron layer): In this study, the number of neurons in the hidden layer was built automatically in SPSS software, the minimum number of neurons was 1, and the maximum number of neurons was 50.
Step 3: ANN training
Network training results will show total leveling errors (SSE), relative errors (RE), and correlation coefficients (R2) of 3 small sets of data divided from set 1, which is the error of the network training dataset, the error of cross-checking, and the network test error.
Step 4: Check the accuracy of the ANN
If the reliability of the network after the test does not achieve the desired results, one of the following two ways will be done:
-
- Keep reheating the network for better results.
-
- Go back to step 2, adjust the number of neurons in the hidden layer or network structure, and then rehearse the network.
A well-functioning ANN will produce the following results:
-
- Network training error, cross-checking, low network testing.
-
- Network training errors in stable final loops.
-
- The degree of over-joint is negligible.
Step 5: Use the ANN to forecast
Based on the sum of the margin error (SSE), Relative error (RE) lowest, and correlation coefficient (R2) to choose the best forecasting model.
3. RESULTS
The total number of dengue cases in the period 2010 - 2020 in Vung Tau City was reported to be 18,441 cases. The highest number of dengue cases was recorded in 2012 (1,879), 2013 (2,884), and a sudden increase in 2019 (4,702 cases) (Figure 1).
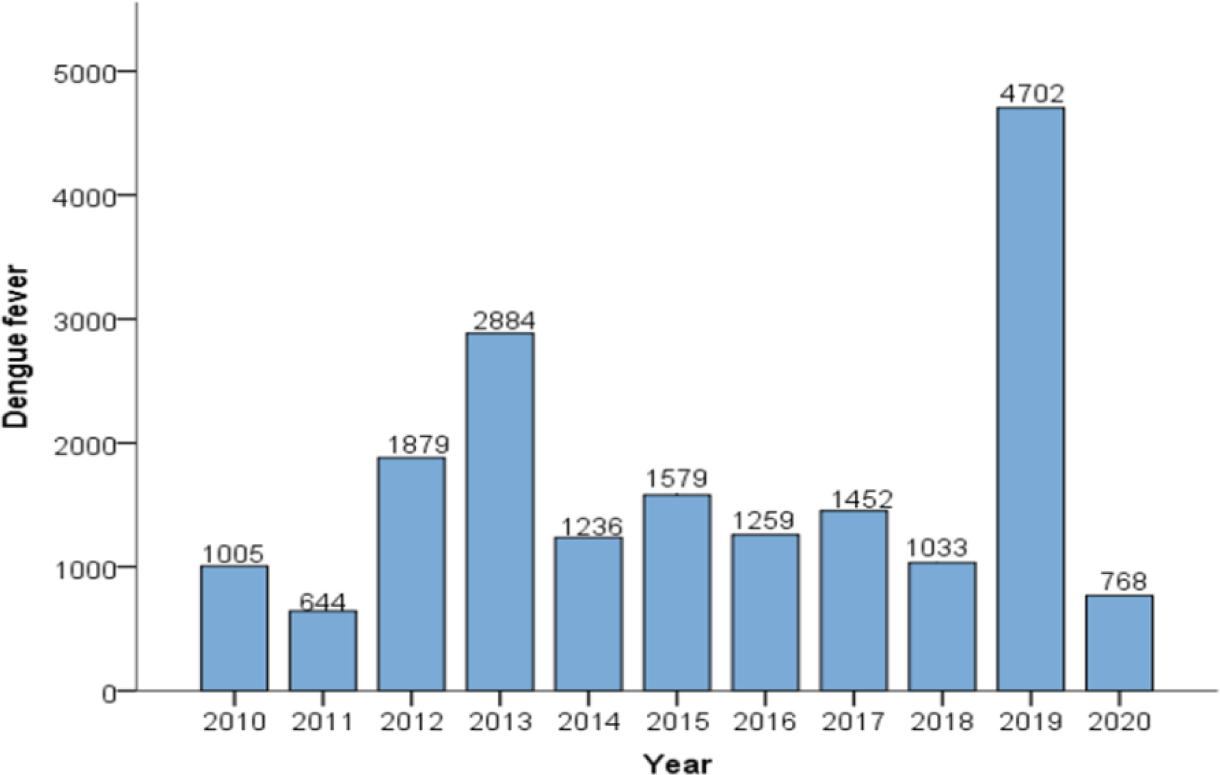
The number of dengue cases tends to be markedly divided seasonally (Figure 2). At the time of the rainy season, between July and September, the average monthly number of dengue cases tends to increase (1483 cases – 2953 cases), peaking at around August (3145 cases). Then, gradually decrease from October to June next year (2622 cases - 1006 cases).
Vung Tau City has a fairly stable average monthly temperature (Figure 3). Specifically, the average temperature tends to rise continuously from February to May (the highest is 30.7°C); gradually decrease from June to January next year (low 25°C).
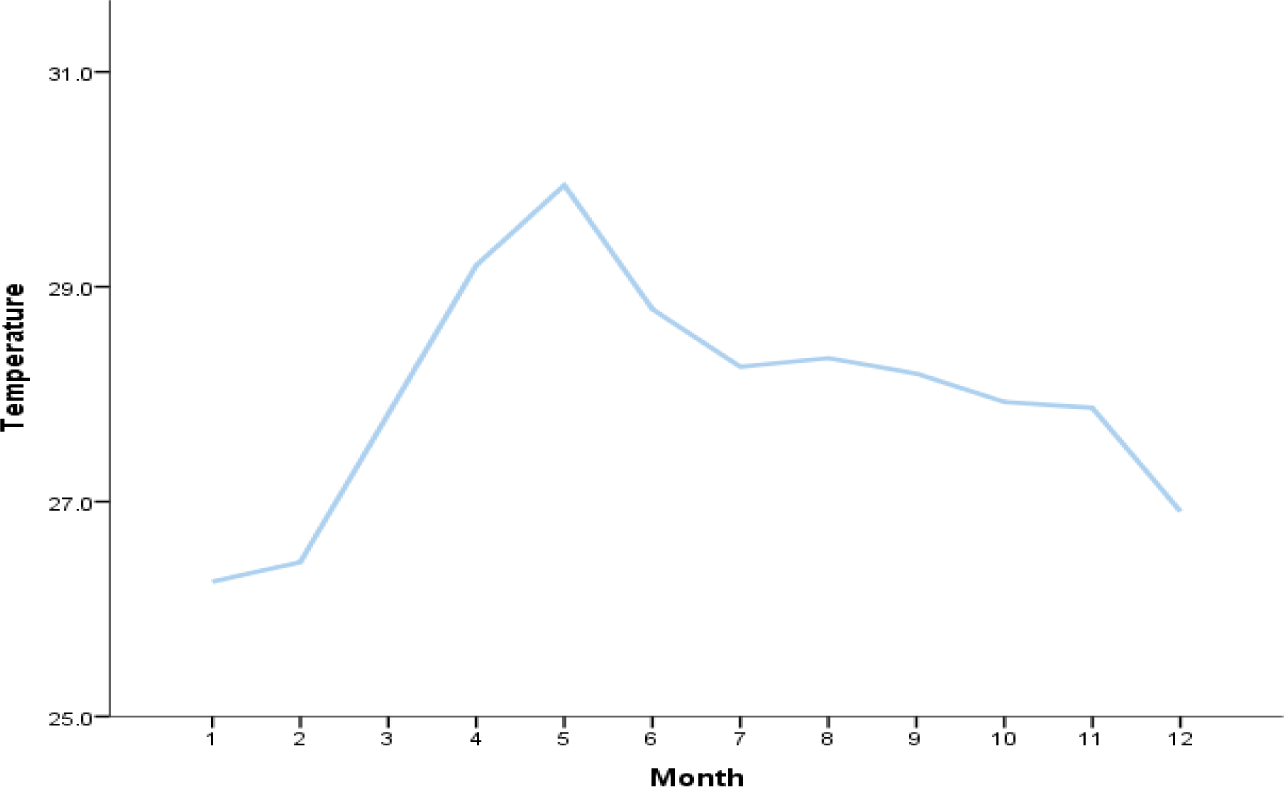
The average monthly rainfall has a marked seasonal divide (Figure 4), the heavy rainy season lasts from March to November (with the highest rainfall of 250 mm), the rainy season is less from December to February next year (the lowest rainfall is 0 mm).
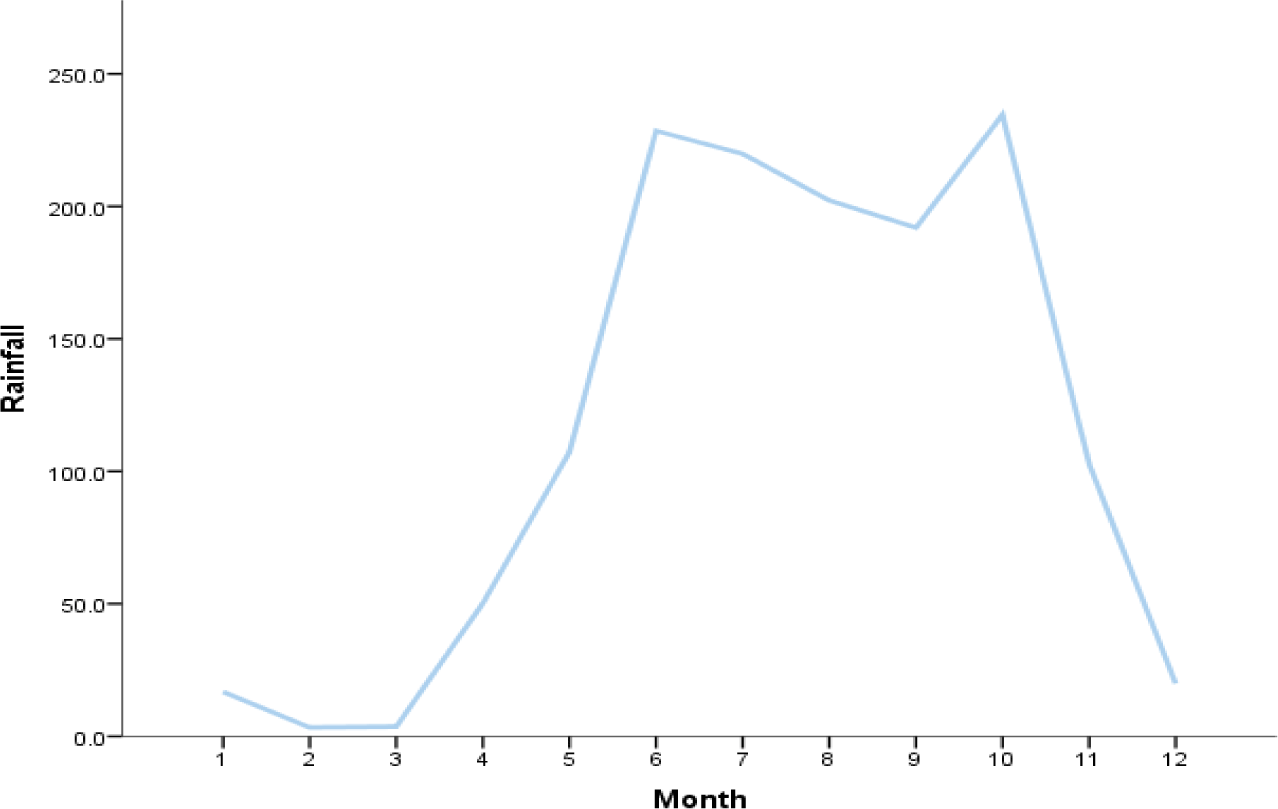
Figure 5 gives results, the average monthly humidity in Vung Tau city is relatively high. High average humidity falls between June and October (from 79% to 82%), around November to May next year there is low average humidity (from 78% to 75%).
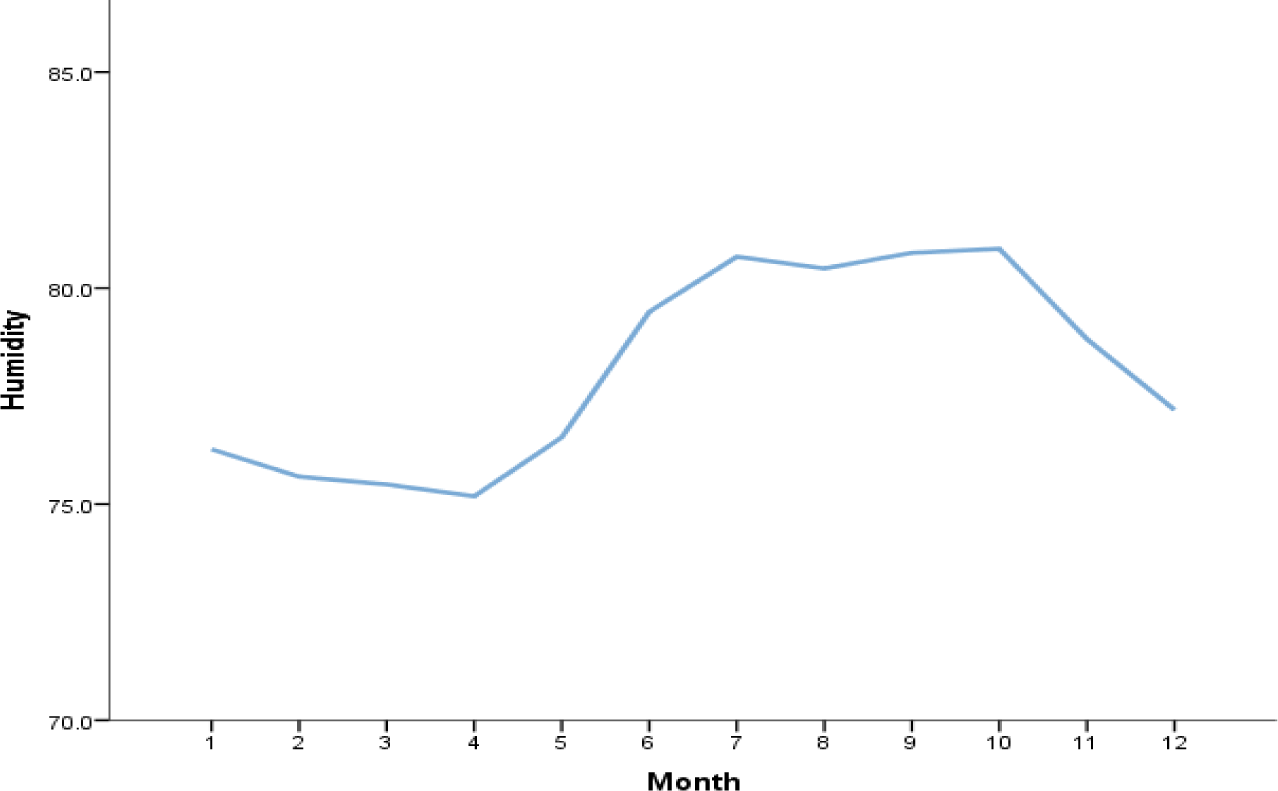
The average monthly wind speed has an uneven distribution (Figure 6). Specifically, the average high wind speed falls between February and August (from 1.9 m/s to 2.2 m/s); the low average wind speed falls between September and January next year (from 1.9 m/s to 1.5 m/s).
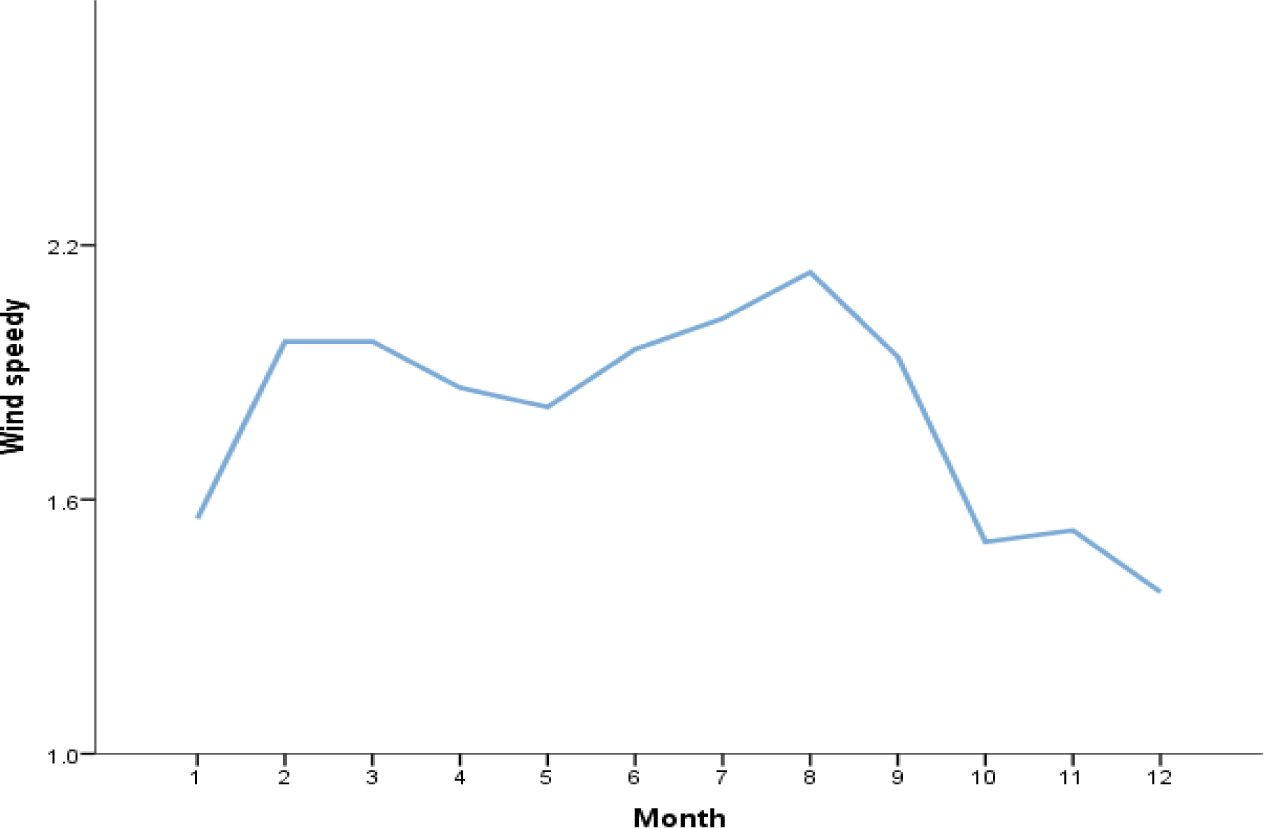
When not considering the lag of weather factors, from Table 1, the number of dengue cases and rainfall are statistically significant positive correlation (r=0.175, p<0.05).
When considering weather factors at a lag-1, the number of dengue cases and rainfall (r=0.354, p<0.001), humidity (r=0.261, p<0.05) were statistically significant positive correlation.
When considering weather factors at a lag-2, the number of dengue cases and temperature (r=335, p<0.001), precipitation (r=0.442, p<0.001), humidity (r=0.227, p<0.001) have a statistically significant positive correlation.
When considering weather factors at a lag-3, the number of cases of DENGUE and temperature (r=0.442, p<0.001), precipitation (r=0.431, p<0.001), humidity (r=0.187, p<0.05), wind speed (r=0.203, p<0.05) have a statistically significant positive correlation.
The input data will be based on the correlation results of the number of dengue cases and weather factors, we have selected the variables that are correlated and statistically significant to include in the model, namely that there are 10 input variables: Temperature (T), Rainfall (R), Humidity (H), Wind speed (WS), Temperature lag-1 (TL1), Rainfall lag-1 (RL1), Humidity lag-1 (HL1), Wind speed lag-1 (WSL1), Temperature lag-2 (TL2), Rainfall lag-2 (RL2), Humidity lag-2 (HL2), Wind speed lag-2 (WSL2), Temperature lag-3 (TL3), Rainfall lag-3 (RL3), Humidity lag-3 (HL3), Wind speed lag-3 (WSL3). The process of building hidden layers for the ANN is a trial and can go wrong. We will change the number of hidden layers and the number of neurons in each hidden layer. After the analysis, we selected the following 7 models (14) (Table 2).
The network training is done using SPSS software, the data consisting of 132 observations, of which 70% is used for training and 30% is used for confirmation sets. This process of division is carried out at random. We compared the forecast results of 7 ANN models based on SSE, RE, R2 criteria.
Results from table 3 show that for the ANN 10-7-1 model training process gives the best results (SSE=25.23; RE=0.58), but the network test is different than the rest of the models; In addition, during the network test, the ANN 10-5-3-1 model has the best SSE results (SSE=10.02), the ANN 10-4-1 model has better RE results than the rest (RE=0.8). In terms of correlation levels, the ANN 10-7-1 model has the highest results of all models (R2=29.1%). Therefore, we choose the ANN 10-7-1 model as a model for forecasting the dengue epidemic in Vung Tau City (Figure 7).
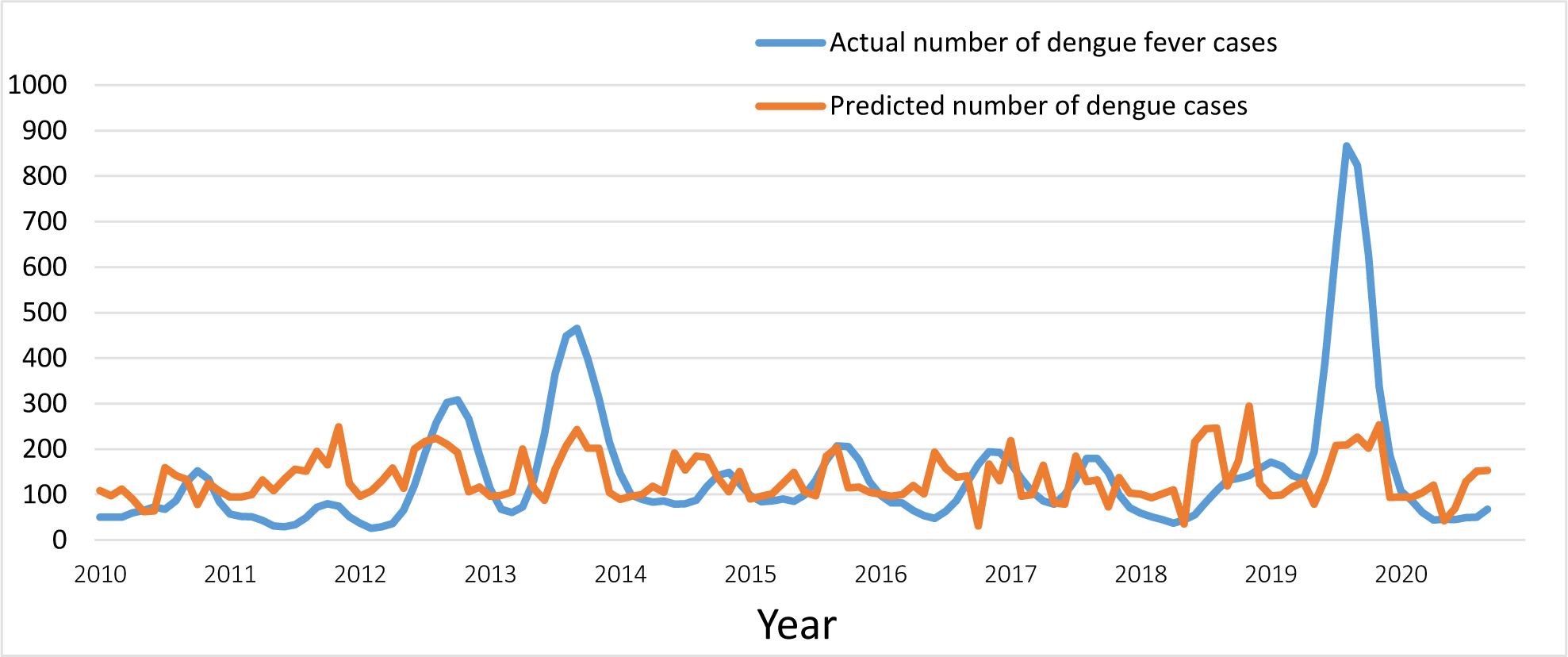
In addition to predicting the number of dengue cases, the ANN model also analyzes the importance of independent variables that impact dependent variables. Specifically, in our study, there are 4 weather factors (temperature, rainfall, humidity, wind velocity) and 3 month lags (lag-1, lag-2, lag-3), The ANN model will analyze which independent variable contributes the most to the model through percentage. According on the results, the rainfall variable has a 100% impact on the dengue case variable, followed by the humidity variable at a 2-month lag has affected about 98% on the dengue case variable, etc., similar to that, temperature variable at a 2-month lag had the least effect on the dengue case variable (about 30%) in the ANN model (Figure 8).
4. DISCUSSION
The number of dengue cases in Vung Tau City increased in 2012, 2013, 2019, especially in 2019, the number of dengue cases increased suddenly. According to Ba Ria - Vung Tau Provincial Preventive Medical Center in 2019, there is an increase in the number of dengue cases with the following causes: the first is the epidemic cycle from 3-5 years and there is the circulation of the virus that causes dengue 2 (previously, dengue is mainly caused by dengue virus 1, so this strain has caused an outbreak of dengue due to the not yet-had community immunity to the dengue virus strain 2 (17). In addition, when the number of dengue cases increases between the middle and the end of the year, weather factors such as wind temperature and speed tend to decrease, rainfall and humidity tend to increase, contributing to facilitating the reproduction and development of mosquitoes. In Australia, one study found that the duration of larval development to maturity is inversely proportional to temperature, ranging from 7.2 ± 0.2 days at 35°C to 39.7°C and 7.2 ± 2.3 days at 15°C, with a maximum survival rate of 88-93% of mosquitoes in the range of 20 to 30°C(18). Another study in Taiwan showed rainfall less than 200 mm (per month) from May to June or less than 100 mm in April, a relatively high risk of dengue(19). The study, conducted in Guangzhou, China, showed that a 1% increase in the relative humidity of a 7-14 day delay was associated with a 1.95% risk of dengue (CI 95%: 1.21% to 2.69%)(20). As such, weather factors (temperature, precipitation, humidity,...) are closely related to the number of dengue cases. The weather changes and the number of dengue cases may change.
The correlation between the number of dengue cases and weather factors: The number of dengue cases is correlated with weather factors (temperature, precipitation, wind speed) but only rainfall is statistically significant with the number of dengue cases (p<0.05). This result is similar to the study in Khanh Hoa, the correlation between the number of dengue cases and rainfall in the same month (7). This may explain that because the life cycle of mosquitoes goes through 4 stages, in the first 3 stages (eggs - stick beetles - mausoleums) mosquitoes all grow in the water, so rainfall can increase the number of mosquitoes by providing more breeding sites (5).
The correlation between the number of dengue cases and weather factors at a 1-month delay: The number of dengue cases is statistically correlated and statistically significant for precipitation and humidity factors (p<0.05), on the other hand, there is no correlation but statistical significance with wind temperature and speed. A study in France showed that the number of dengue cases was correlated with humidity and temperature, but did not correlate with rainfall(21). Also in Khanh Hoa, the author did not find the impact of wind speed on the number of dengue cases (7). Under favorable weather conditions, after only about 10-15 days, from eggs, mosquitoes, will develop into sticks, mausoleums, young mosquitoes and become adult mosquitoes, female mosquitoes after hatching from eggs only about 5-8 days later have become adult mosquitoes and can suck blood (sting) humans(22). Therefore, the delay of 1 month is suitable for the life cycle of dengue mosquitoes.
The association between the number of dengue cases and weather factors at a 2-month delay: When considering whether factors at a 2-month delay, the number of dengue cases is correlated favorably with all-weather factors, but only not statistically significant for wind speed factors (p>0.05). At the same time, the study in Khanh Hoa author pointed out the correlation of rainfall factors, humidity, wind speed; however, it is not correlated with temperature (7). Another study in Barbados found that temperatures at a 12-week delay (about two months) were strongly correlated with the number of dengue cases(23). Thus when the rain lasts, leading to high rainfall and humidity, low temperature, stable wind speed favorable for mosquitoes to grow.
The association between the number of dengue cases and weather factors at a 3-month delay: The number of dengue cases is correlated statistically and statistically for all-weather factors (p<0.05). A study by author Hanh Thi Tuyet Tran and colleagues conducted in Hanoi also showed the correlation of weather factors (temperature, precipitation, humidity) at a delay of 3 months, specifically when the temperature increased by 1°C, the number of dengue cases increased by 23.48%, humidity increased by 1%, increased by 7.97% in the number of dengue cases, rainfall increased by 1mm, the number of dengue cases also increased by 3.96%(24). At the same time, in Khanh Hoa, the author also pointed out the largest correlation of wind speed when the impact is 3 months late, when the average monthly wind speed increases by 1 m/s, the risk of dengue in the next 3 months will be reduced to 0.73 times (p =0.005; CI 95%: 0.58 - 0.90) (7). Perhaps, this is the most appropriate time for mosquito growth and the transmission of dengue, so this can also be an important stage for intervention in dengue prevention measures.
Of all the models we analyzed, the one with the best forecast result was the ANN 10-7-1 model (SSE=25.23; RE=0,58; R2=29.1%). Similarly, a study conducted in Thailand also used the ANN to forecast dengue outbreaks to produce more than 80% accurate forecast results, but they used the average localized error index (MSE= -1.77) to evaluate the model(25). HM. Aburas and his colleagues’ artificial neural network forecasting model, which also gives RMSE=50.7 and a correlation coefficient of 0.91(9). From there, our research can be applied in practice to predict the future number of dengue cases in Vung Tau City.
In addition, our study has the following limitations: firstly, the sudden increase in dengue cases in 2019 can cause the forecasting process to be affected, leading to the predicted data being much different from the actual data, Therefore, in the future, it is necessary but the study re-examines the accuracy of the model. Second, the study only predicted the number of dengue cases relatively accurately and the upward trend - a steady, not stable decline compared to the actual number of dengue cases. Third, the study only used weather factors that other factors such as housing index, mosquito density, the population in the study area,... Not being included may affect the number of dengue cases. Finally, the 132-month (11-year) data corresponds to only two cycles of the outbreak, which is not large enough to better predict the number of dengue cases.
Conclusion
In summary, the results of the analysis using the artificial neural network model (ANN) showed that the ANN 10-7-1 model forecasted better than the rest of the ANN models. However, the study only predicted the number of dengue cases relatively accurately, so we recommended other similar studies to compare the model’s predictive results before applying them in practice. At the same time combined with factors such as housing index, mosquito density, population,...to find out the link to the number of dengue cases, thereby building a more effective prediction model.